Abrazolica
Home     Archive     Tags     About        RSS
I don’t watch a lot of football but this year I did watch Super Bowl LVIII between the San Francisco 49ers and the Kansas City Chiefs played February 11 2024 in Las Vegas NV . I think it may be the best football game I’ve ever seen. The final score was Kansas City 25, San Francisco 22 in overtime.
If you didn’t have the chance to watch the game is there anything the score by itself can tell you about how the game was played? In American football, a team can score in the following ways:
- 6 points is a touchdown
- 7 points is a touchdown with 1 point conversion
- 8 points is a touchdown with 2 point conversion
- 3 points is a field goal
- 2 points is a safety
Touchdowns with 2 point conversions and safeties are rare. If we exclude those then how many ways can a team end up with 25 or 22 points? This amounts to finding all the integer partitions of 25 and 22 using the parts (3,6,7). For 25 there are 4 possible partitions: 3+3+3+3+3+3+7, 3+3+3+3+6+7, 3+3+6+6+7, 6+6+6+7. For 22 there are 3 possible partitions: 3+3+3+3+3+7, 3+3+3+6+7, 3+6+6+7.
If we’re not interested in the order that the points were scored then the number of ways the final score can be 25 to 22 is number of partitions of 25 times the number of partitions of 22 or 4*3=12. If we are interested in the order of the points then we have to look at how many ways each partition could have occurred.
For the partitions of 25 we have:
- multiplicity(3+3+3+3+3+3+7)=7!/6!=7
- multiplicity(3+3+3+3+6+7)=6!/4!=30
- multiplicity(3+3+6+6+7)=5!/(2! 2!)=30
- multiplicity(6+6+6+7)=4!/3!=4
For the partitions of 22 we have:
- multiplicity(3+3+3+3+3+7)=6!/5!=6
- multiplicity(3+3+3+6+7)=5!/3!=20
- multiplicity(3+6+6+7)=4!/2!=12
The total number of ways the points could have occurred is then
(7 + 30 + 30 + 4) * (6 + 20 + 12) = 71*38 = 2698
The actual way the points were scored, including the order is
- Kansas City: (3,3,7,3,3,6)
- San Francisco: (3,7,6,3,3)
It is interesting that both of these ways come from partitions with high multiplicities. You could define a probability distribution over the partitions based on their multiplicities. For the partitions of 25 the probabilities are:
- probability(3+3+3+3+3+3+7)=7/71=0.098
- probability(3+3+3+3+6+7)=30/71=0.423
- probability(3+3+6+6+7)=30/71=0.423
- probability(6+6+6+7)=4/71=0.056
For the partitions of 22 the probabilities are:
- probability(3+3+3+3+3+7)=6/38=3/19=0.158
- probability(3+3+3+6+7)=20/38=10/19=0.526
- probability(3+6+6+7)=12/38=6/19=0.316
The Kansas City score came from a partition group with probability 0.423 and the San Francisco score came from a partition group with probability 0.526.
This kind of analysis is possible because of the different ways a team can score in American football. The score contains more information than it does in a sport like soccer where the score is only accumulated in one point increments. With more information you can narrow down the number of games even more. Just knowing that the game ended in overtime and knowing the rules of overtime play should narrow it down. Better yet would be knowing the score at the end of each quarter.
In our Creating Noise book we show how to use RC ladder networks to create pink noise. An \(n^{th}\) order network is shown below.
The R and C values are given by the following (equations 37 and 38 in the book).
\[C(n,i) = \frac{4i-1}{(2(n-i)+1)16^i}\frac{\binom{2(n+i)}{n+i}}{\binom{2(n-i)}{n-i}}\] \[R(n,i) = \frac{(4i-3)(2(n-i)+1)16^i}{4(n-i+1)(n+i)}\frac{\binom{2(n-i)}{n-i}}{\binom{2(n+i)}{n+i}}\]
Using these equations is a bit cumbersome so here are some asymptotic versions of the equations which will go into the next edition of the book.
\[C(n,i) = \frac{4i-1}{(2(n-i)+1)}\sqrt{\frac{n-i}{n+i}}\] \[R(n,i) = \frac{(4i-3)(2(n-i)+1)}{4(n-i+1)\sqrt{(n+i)(n-i)}}\] \[C(n,i)R(n,i) = \frac{(4i-1)(4i-3)}{4(n-i+1)(n+i)}\]
These equations obviously only work for \(i<n\). For \(i=n\) the asymptotic equations are
\[C(n,n) = \frac{4n-1}{\sqrt{2\pi n}}\] \[R(n,n) = \frac{(4n-3)\sqrt{2\pi n}}{8n}\]
The last resistor in the chain has the asymptotic formula \[R_L(n)=\sqrt{2\pi n}\]
In the limit \(n\rightarrow\infty\) with \(i/n\rightarrow x\) both the R and C equations have the following form
\[\frac{2x}{\sqrt{1-x^2}}\]
where \(x\) has the range \(0<x<1\). In this limit the ladder becomes a continuous transmission line and the total capacitance or resistance (measured from the beginning of the line) is found by integrating the above equation
\[C(x)=R(x)=2\left(1-\sqrt{1-x^2}\right)\]
In the bridge circuit shown below, find the value of the resistance \(c\) such that the equivalent resistance connected across the voltage source is also equal to \(c\). Show that for this value of \(c\), the voltage across \(c\) is equal to \((\sqrt{b}-\sqrt{a})/(\sqrt{b}+\sqrt{a})\). Try to solve this problem yourself before looking at the solution below.
Start by finding the equivalent resistance. The easiest way to do this is to replace the voltage source with a current source \(I\), and then find the voltage \(V_1\) at node 1. The equivalent resistance is then \(V_1/I\). This involves solving 3 equations for nodes 1, 2 and 3. The equations are Kirchoff’s current law applied to the nodes. Leaving out the details, the equivalent resistance is
\[R = \frac{2ab+(a+b)c}{a+b+2c}\]
If you set \(R=c\) and solve for \(c\), you get \(c=\sqrt{ab}\). In other words, when \(c\) is equal to the geometric mean of \(a\) and \(b\), the equivalent resistance is equal to \(c\).
To solve the second part of the problem we need an expression for the voltage across \(c\). Going back to the voltage source and writing the node equations for nodes 2 and 3, we get
\[(1/a + 1/b + 1/c)V_2 - (1/c)V_3 = (1/a)V_1\]
\[-(1/c)V_2 + (1/a + 1/b + 1/c)V_3 = (1/b)V_1\]
Solving these 2 equations for \(V_2/V_1\) and \(V_3/V_1\) we get the following expression for the voltage across resistor \(c\).
\[\frac{V_2-V_3}{V_1} = \frac{(b-a)c}{(b+a)c+2ab}\]
If you substitute the value \(c=\sqrt{ab}\) into this equation, you find that
\[\frac{V_2-V_3}{V_1} = \frac{\sqrt{b}-\sqrt{a}}{\sqrt{b}+\sqrt{a}}\]
This simple circuit can calculate the ratio of the sum and difference of the square roots of 2 numbers.
Sometimes you have to measure a voltage that has a high output impedance. To do this accurately you need to know the input impedance of your voltmeter. Ideally it should be much much larger than the impedance of what you’re trying to measure. The following figure illustrates the situation.
The voltage we’re trying to measure is \(V_x\) and it has an output resistance of \(R_x\). The meter has an input resistance of \(R_m\). The voltage measured by the meter will then be
\[V = \frac{V_xR_m}{R_x+R_m} = \frac{V_x}{1+R_x/R_m}\]
So you can see that \(V\approx V_x\) only when \(R_m>>R_x\). If you happen to know the values of \(R_x\) and \(R_m\) then you can determine what \(V_x\) is even when the two resistances are of the same magnitude. How do you measure the input resistance of your voltmeter? If you apply a known \(V_x\) using a known \(R_x\) then you can solve for \(R_m\) in the above equation.
\[R_m = \frac{R_x}{\frac{V_x}{V}-1}\]
We did this for the old Scope multimeter you see in the following picture using \(V_x=12v\), \(R_x=1M\Omega\).

The meter read \(10.98v\) which gave us \(R_m=10M\Omega\). This is pretty typical for most handheld multimeters.
Some really cheap multimeters such as the one shown below that we got at Harbor Freight Tools showed an input resistance of only \(1M\Omega\). You have to be careful about what you measure with a meter like that. In some cases it can give you very inaccurate readings.
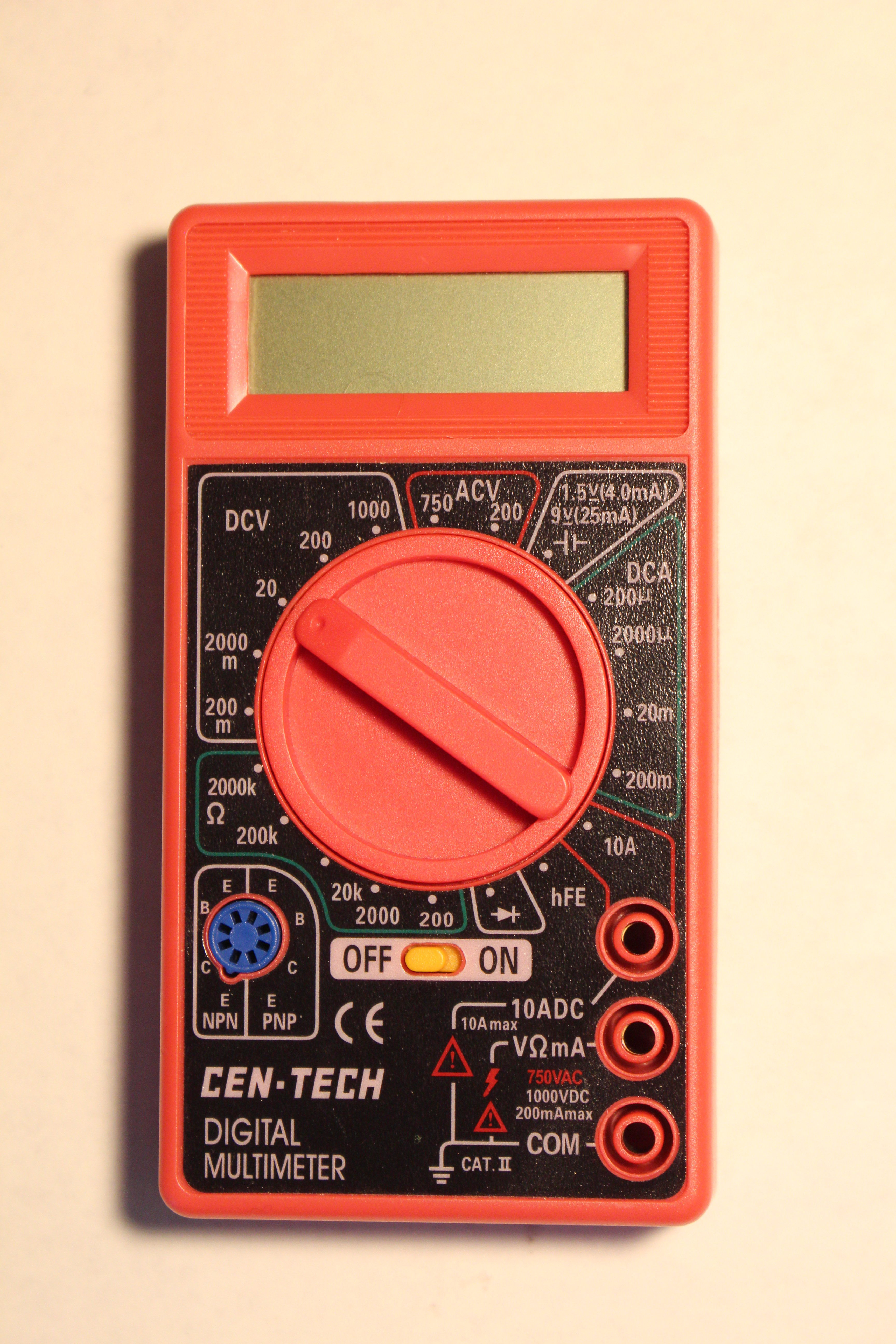
© 2010-2024 Stefan Hollos and Richard Hollos